Deep Learning Microscope for Slide-Free and Digital Histology
Funding Agency:
NIH / National Institute of Dental & Craniofacial Research
Collaborators:
Ashutosh Sabharwal ashu@rice.edu (Principal Investigator), Rebecca Richards-Kortum (Co-Principal Investigator), Lin Zhong (Co-Principal Investigator), Richard Baraniuk (Co-Principal Investigator), Ashok Veeraraghavan (Co-Principal Investigator)
Overview:
Anatomic histopathology plays a central role in disease diagnosis and in surgical procedure guidance to ensure delivery of quality healthcare and treatment. At the time of surgery, for example, tumor margins are ideally assessed with fast frozen section pathology to help ensure complete tumor resection while sparing normal tissue. Unfortunately, the time- and labor-intensive slide preparation process requires expensive equipment and specialized personnel, so it is not widely available in many settings including the rural US; even in settings with the clinical infrastructure to perform frozen section, only a small fraction of the margin is manually examined. In resource-limited global settings, a dire shortage of pathologists makes it more challenging to provide routine diagnostic pathology. Therefore, there is a critical need for affordable tools to support quality histopathology programs throughout the world. The goal of this proposal is to use recent advances in optical fabrication and artificial intelligence to develop a new and affordable tool, the deep learning extended depth-of-field (DeepDOF) platform, to rapidly examine fresh tissue resections without extensive slide preparation, while providing computer-aided image analysis at the point of care. We will demonstrate and validate its use for tumor margin assessment in patients with oral squamous cell carcinoma, the sixth most common malignancy worldwide.
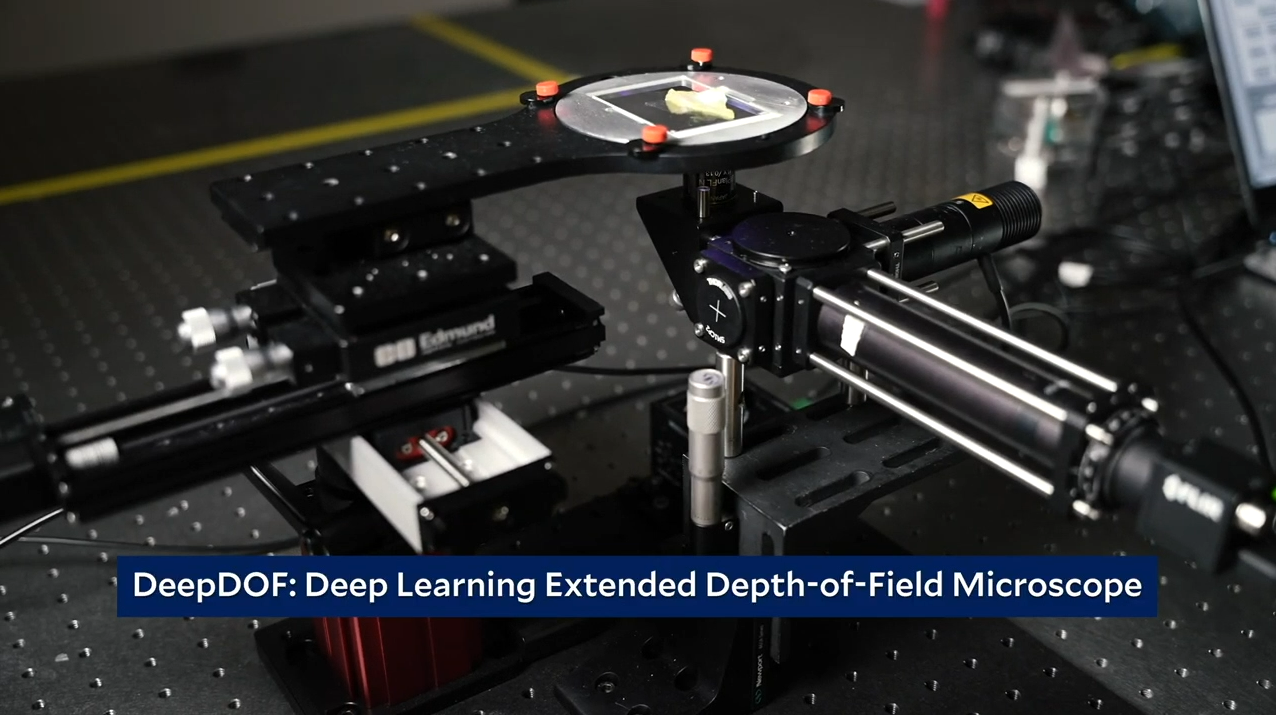